The State of SciML in the Real World
By on 24 Jan 2025TL;DR
Recap of the inaugural workshop on the industrialization of Scientific Machine Learning, co-organized with and hosted by The Institute for Computational and Experimental Research in Mathematics at Brown University.This was originally posted on 4 April 2024, on Pasteur Labs' LinkedIn page.
Two weeks ago we gathered nearly 100 scientists from around the world to Brown University, for a weekend of rich dialogue and cutting-edge advances in the industrialization of Scientific Machine Learning (SciML). This first-of-its-kind workshop, co-organized by Pasteur Labs and hosted by ICERM, brought together the Industrial-SciML world-leading players working at the forefront of advancing R&D who are armed with the critical experience necessary for building and scaling SciML in the wild1.
The invited speakers ranged from Siemens and Ansys directors on digital engineering, to NASA and national lab PIs on Earth and space systems, and of course startups like Pasteur Labs on transformational technologies. If you missed this opportunity or want to relive the sessions, the recorded talks and follow-up discussions are now posted on the workshop website.
”The workshop was incredibly valuable as it provided insight into the forefront of machine learning applied to scientific problems. Witnessing the diverse approaches showcased was eye-opening... I'm completely confident that SciML will see substantial growth in industry pipelines within the next two years. The convergence of machine learning and scientific domains is poised to revolutionize various sectors. The synergy between these fields enables more efficient problem-solving and fosters innovation, making it inevitable for their integration into industry pipelines. Beyond the invaluable insights gained, [the Pasteur Labs event] provided a platform to connect with like-minded individuals, and the potential for collaboration with individuals boasting extraordinary expertise was truly inspiring, all making the workshop an unforgettable experience.”
— Edison Vazquez, Global Data Science Leader, Schneider Electric
Why Now?
While recent years have shown immense research progress in accelerating classical physics solvers and discovering new governing laws for complex physical systems, SciML methods and applications fall short of real-world utility in essentially all domains. Across all physical and life sciences, from small scales like precision medicine to large industrial energy systems, the technical maturity and validations necessary are lacking, failing, or unknown to the world of SciML research.
In the context of traditional and advanced industrial settings, the adoption of SciML requires operating in digital-physical environments governed by large-scale, three-dimensional, multi-modal data streams that are confounded with noise, sparsity, irregularities and other complexities that are common with machines and sensors interacting with the real, physical world.
We believe that it is now the time to upgrade academic SciML to the industrial world by stress testing these techniques on real-world applications.
"The Pasteur Labs @ ICERM workshop was a valuable venue for industry professionals to learn and discuss state-of-the-art methods, and bridge the gap in technology readiness levels across fundamental research, applied research and technology development."
— Balaji Jayaraman, Senior Scientist, GE Research
And What Happened Exactly?
Through engaging talks and coffee-break discussions, the workshop highlighted the most outstanding challenges and bottlenecks in applying SciML to industrial contexts, showcased the latest breakthroughs, and provided the foundations for near-future achievements and long-term advancements of this widely-valuable domain. Among the topics we heard about during this past weekend, some shined:
The validation of fast and flexible surrogates for multi-physics and multiscale problems in real settings.
The implementation of data-oriented architectures for properly running SciML models and tools in efficient coordination with online data streams.
The design of efficient, robust, and reliable optimization tools for inverse engineering — from optimal design to system identification.
The requirements of cause-effect modeling in industrial and related settings, and if/how SciML plays a role.
The effective detection of causality patterns in industrial data streams (with and without injecting domain knowledge), and the subsequent utility in industrial planning and decision-making (i.e. decision intelligence).
The robust prediction of anomalies and system downtime/failures with probabilistic guarantees, including root-cause analysis.
Jordan Jalving, a Pasteur Labs research engineer (in the picture below), covered all the topics above in one talk, highlighting Pasteur Labs’ breadth of mission and emphasis on real-world validations — his slides and recorded video are now available online.
It was very valuable to see where everybody is. In a setting like this (small, with powerful researchers around) companies like ours tend to talk a bit more openly. Clearly still not in a quite open academic sense – that will likely never happen. Since the ChatGPT announcements in late 2022 the awareness and 'What can AI do for me?' has brought up a lot of interest/activities... Companies can be very different when it comes to the expected accuracy and cost of training — requests vary wildly... The level of education in our customers on SciML particularly for surrogates has gone up significantly over the past 12 months. These requests are exceeding the requests related to Generative Design (as in “draw my car”). In many of the engineering companies we work with, these people reside still with the Research groups, Advanced Methods groups or Optimization groups—their PhDs have switched modeling and simulation (M&S) efforts to SciML.
— Victor Oancea, Sr. Technology Director, Dassault Systèmes
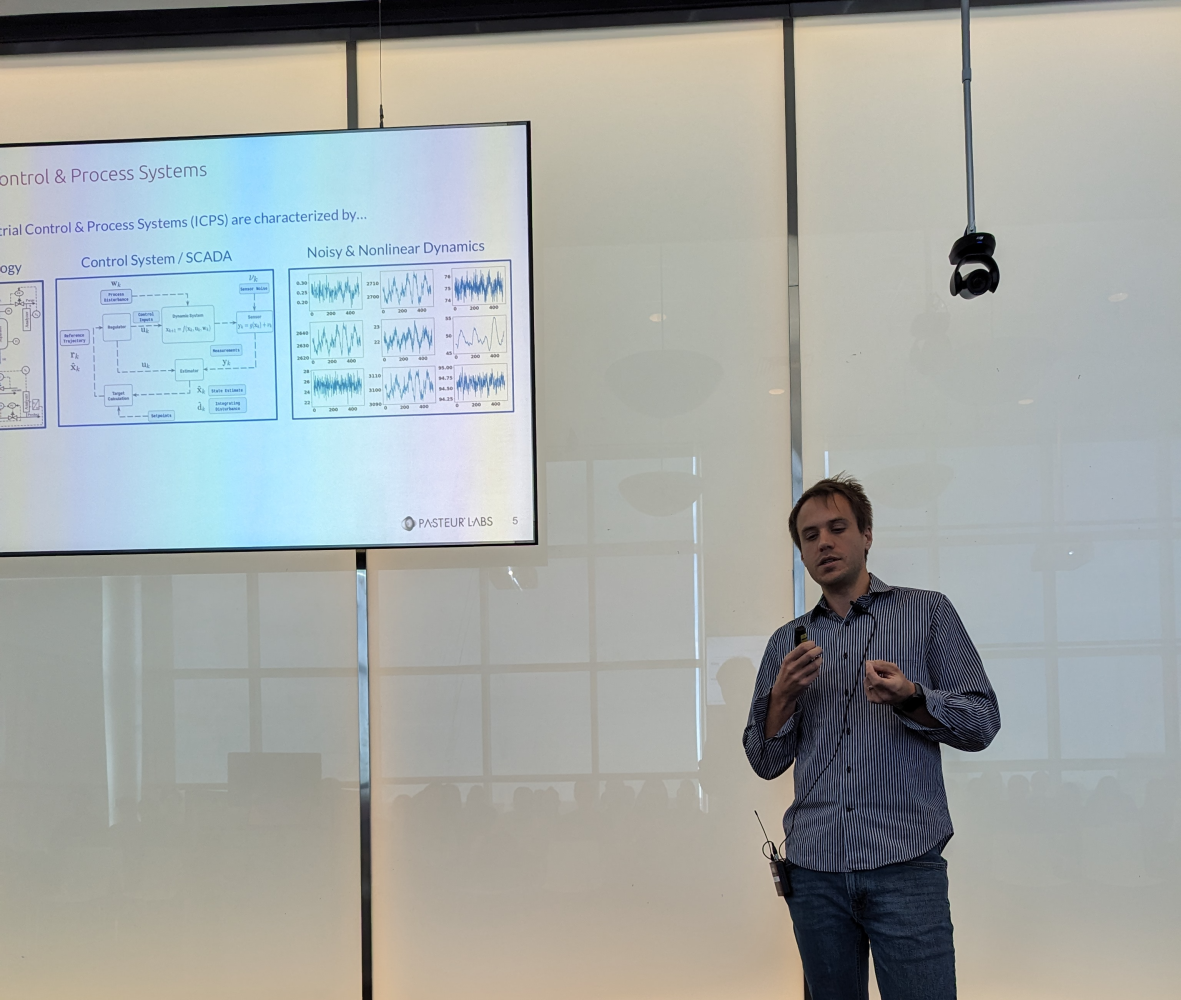
What Did We Learn?
Several speakers highlighted much needed SciML features that are rarely addressed in academic papers but become fundamental in industrial settings.
For industrial online environments, prediction speed and reliability are paramount and are prioritized over accuracy. Our communities should explore and validate SciML solutions that are fast and robust. At the same time, industrial systems may undervalue predictive SciML solutions that deal with longer timescales such as their use in planning and control. Our communities should work together to co-develop these solutions.
Incorporation and/or integration of SciML solutions within existing code-bases and frameworks is key to market adoption. End users do not want to abandon their legacy products.
Development and disclosure of appropriate validation benchmarks is crucial for SciML success in industry. However, this is a challenging task because validation efforts are not incentivized by the current status quo. Having engineering “standards” just like in other disciplines (e.g., mechanical engineering) should be the first step in this direction.
Who Was There?
A key aspect of this past weekend was the striking diversity of the speakers and the audience that motivated and triggered constructive interactions and collaborations. Our speakers (spanning industrial and academic institutions, and with backgrounds in ML and AI, scientific computing, simulation intelligence, and scientific and industrial software) provided unparalleled insights spanning every aspect of applying SciML to real world contexts: from the design of fast emulators that preserve physical properties to the implementation of effective optimization tools for their training, and the critical verification-validation schemes necessary for SciML deployments. And meeting those insights were the rich perspectives of the “end users” of industrial SciML, such as representatives of companies whose pipelines & teams would greatly benefit from the tools discussed during the workshop.
The discourse we fostered will not only pay dividends for the industrial end-users, but it will provide invaluable direction for the SciML developers and researchers to target true challenges with practical solutions and measurable impact. The results will help guide the development of industrial SciML tools and R&D directions for the broader field. Last but certainly not least, the presence of academics was fundamental not only because of the theoretical intuitions and R&D advances they bring, but also because they gained opportunity to learn about industrial needs (and the corresponding SciML research shortcomings) that will inform and shape graduate courses, PhD theses, or even larger funding programs.
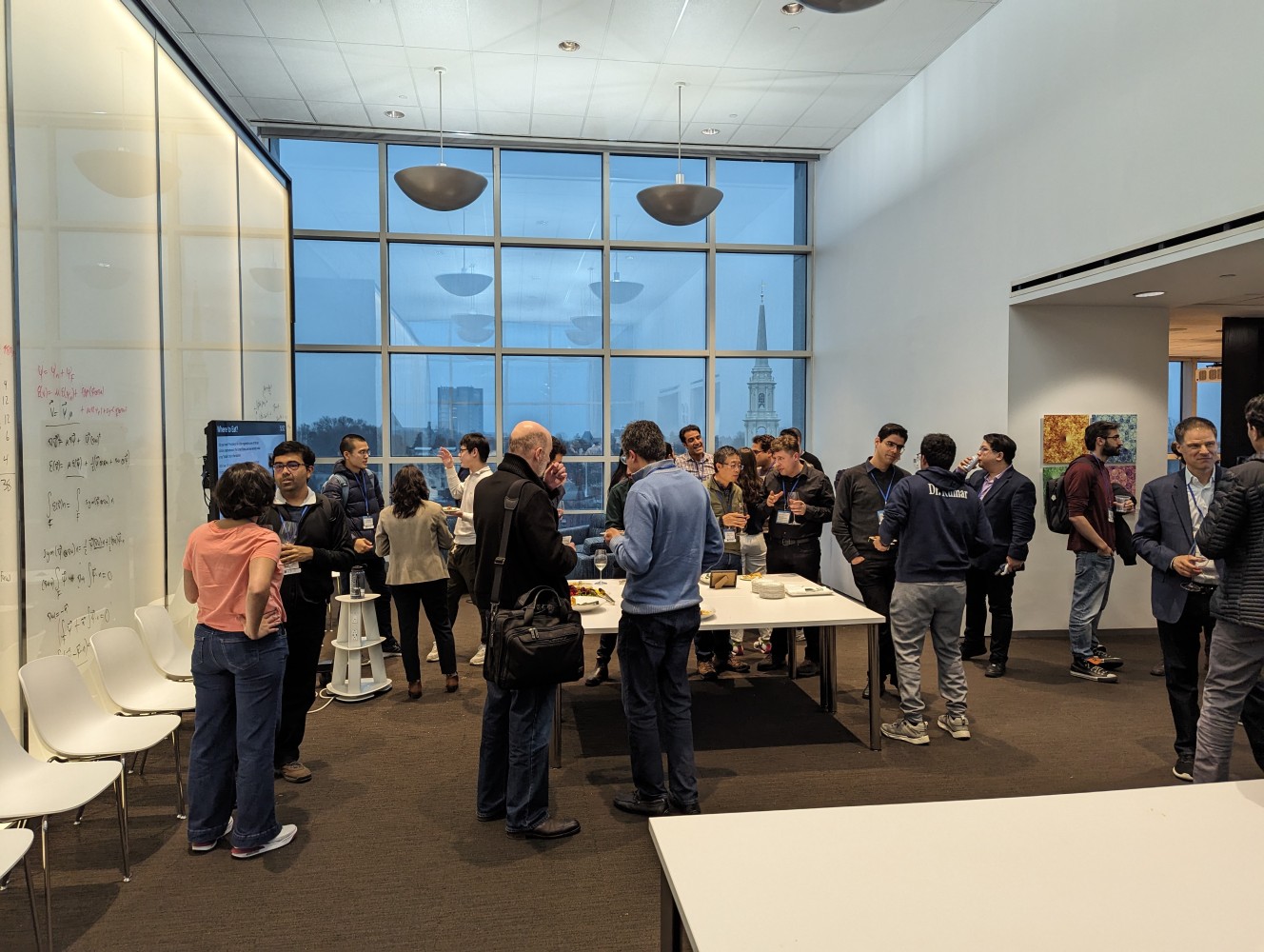
"The adoption of SciML in engineering applications over the next few years will require consistent dialog and alignment between industrial and academic institutions with a clear exchange of expectations, objectives, methodologies and solutions. The Pasteur Labs workshop provided a great opportunity to learn about the SciML research and perspectives in the academic community and opened doors for an open dialogue, important for fostering collaborations and partnerships, between industry and academic researchers."
— Rishikesh Ranade, Lead Researcher - Machine Learning, ANSYS
“[The workshop] helped me understand state-of-the-art in the non-academic space. Interaction with several other companies could foster joint activities in the future. [The workshop had] excellent talks, management, and execution. I especially liked the fact that this workshop was organized over one weekend. Both AI and SciML are growing significantly in the industry."
— Anirban Chandra, Computational Scientist, Shell
Was it a Success?
During the weekend we could feel general vibes of excitement and urge to talk science, implement groundbreaking code, and innovate. We all agreed that this gathering was very much needed and that we should make it a regular check-in. It doesn’t matter if we are competing or collaborating – both bring motivation and stimulate going beyond what we believe is possible. Was it a success then? Well the quotes speak for themselves.
"Overall the value of the workshop was enormous for us. I met five or six people who were willing to share their requirements for SciML products that will be very valuable to the companies we are launching. I gained a greater appreciation for the range of applications, everything from cardiac modeling to container ships and bridges (unfortunately super relevant today…). Having been exposed to the work showcased here, it’s hard to imagine how it’s not relevant to industry pipelines – a matter of how to convert the unconverted as you’re no doubt experiencing at Pasteur Labs.”
— Bob Chatham, Slater Technology Fund
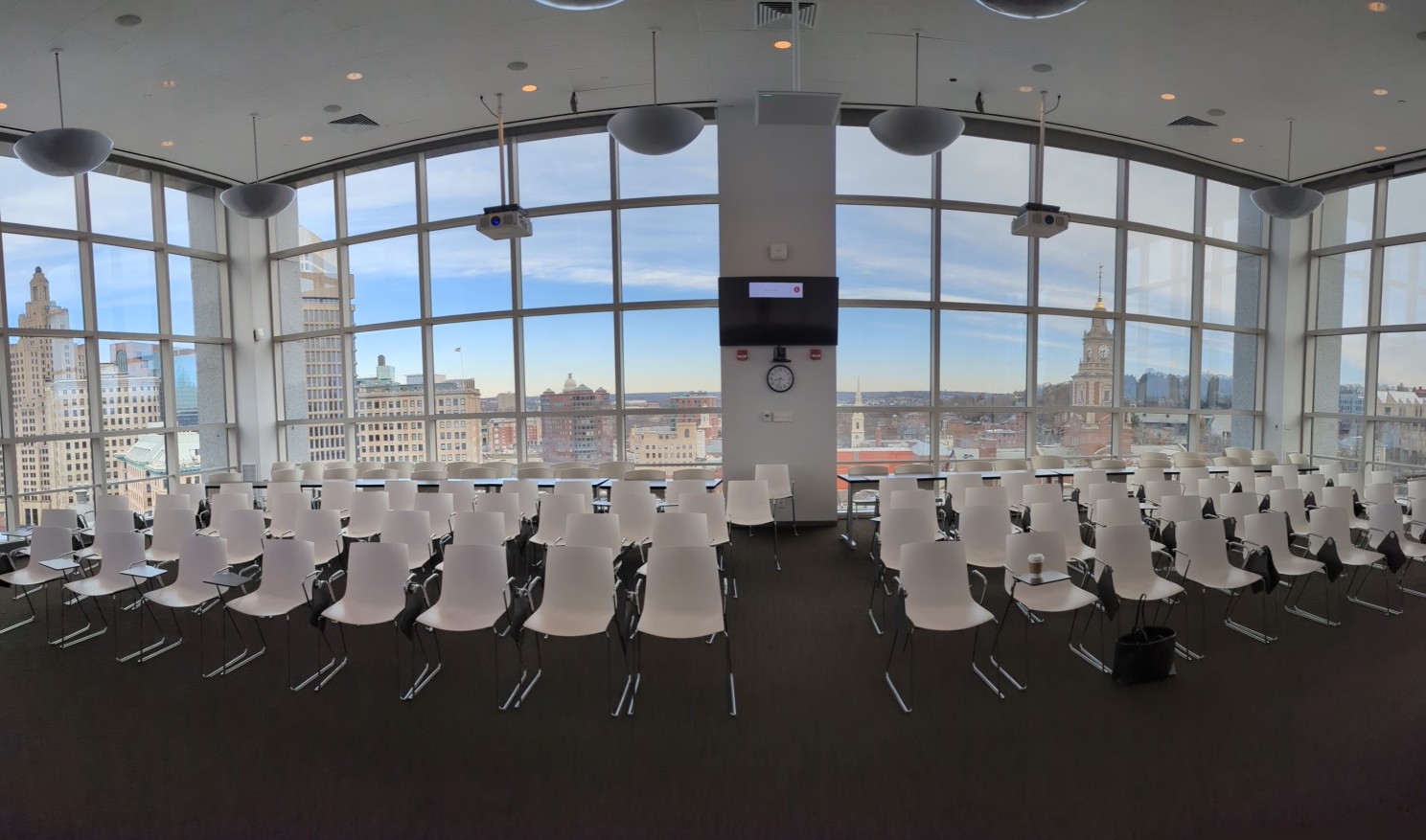
Footnotes
-
For the non-experienced reader, "scientific machine learning" (SciML) is a merger of computational sciences and data-driven machine learning, implemented in software as a set of abstractions in order to leverage existing domain knowledge and physics models within gradient-based learning schemes and accelerated computing platforms. SciML aims at developing new methods for scalable, domain-aware, robust, reliable, and interpretable learning and data-analysis techniques. SciML applies to many systems at all scales, from particle physics and quantum chemistry, to energy and transportation systems, to economics and healthcare, to climate and cosmology. ↩